The Emergence of Small Language Models (SLMs)
The Shift to SLMs:
- Present the idea of SLMs, underlining their developing significance.
- Frame the variables driving this shift, like the requirement for more supportable and open man-made intelligence arrangements.
Historical Context:
- Make sense of how generally, enormous language models (LLMs) have driven the way in man-made intelligence progressions.
- Talk about the underlying leap forwards and the huge achievements accomplished by LLMs.
Key Drivers:
- Detail the key drivers behind the emergence of SLMs:
- Cost Efficiency: SLMs require less monetary venture for preparing and sending.
- Energy Efficiency: They consume essentially less power, tending to natural worries.
- Accessibility: SLMs even the odds, permitting more modest elements to partake in artificial intelligence advancement.
Case Studies:
- Present contextual investigations of fruitful SLMs, delineating their effect and potential.
- Feature how these models are being coordinated into different ventures and applications.
Future Outlook:
- Examine the possible future advancements in SLMs.
- Guess on how they could proceed to develop and impact the more extensive computer based intelligence field.
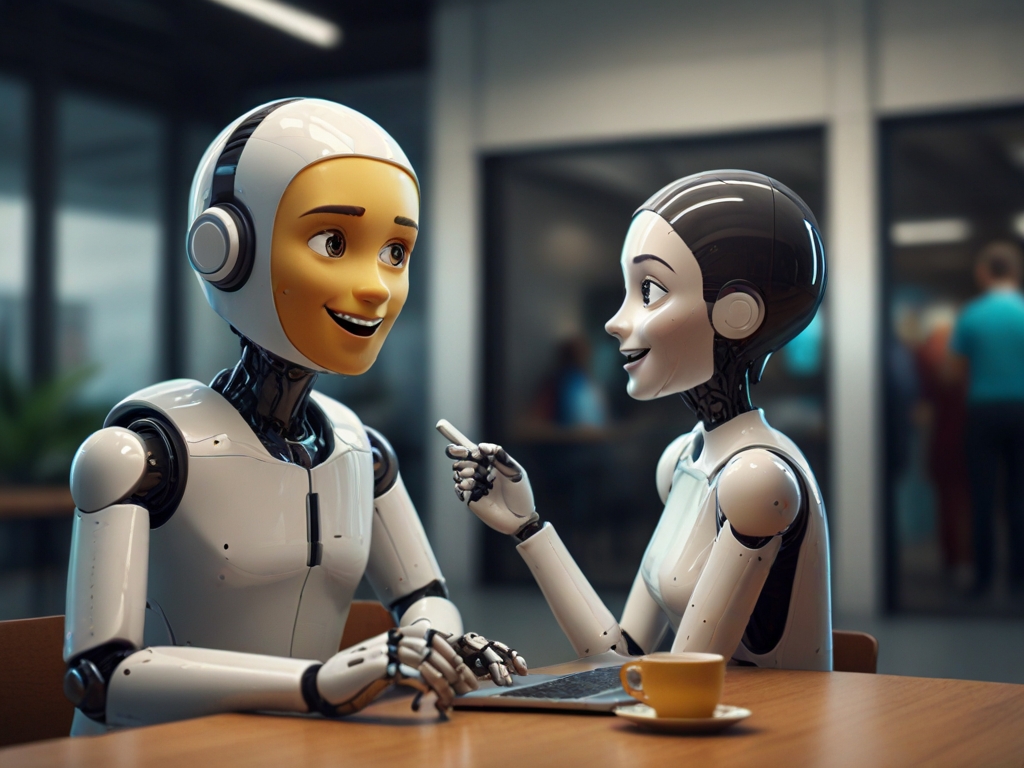
Advantages of SLMs
Cost-Effectiveness:
- Cost Savings: Accentuate how SLMs are more spending plan agreeable, lessening the monetary obstruction to section for computer based intelligence innovative work.
- Resource Allocation: Talk about how the saved assets can be diverted to different areas of development.
Efficiency:
- Faster Training Times: Feature the decreased time expected to prepare SLMs, considering faster emphasess and advancements.
- Lower Energy Consumption: Direct out the ecological advantages of SLMs due toward their lower power necessities.
Accessibility:
- Democratization of AI: Make sense of how SLMs make simulated intelligence innovation more open to a more extensive scope of designers, including specialists and more modest associations.
- Community Growth: Examine the potential for a more dynamic and various open-source local area because of the availability of SLMs.
Adaptability:
- Flexibility in Use: Represent how SLMs can be effortlessly adjusted for different applications, from cell phones to edge processing.
- Customization: Discuss the simplicity of tweaking SLMs for explicit errands or dialects, which is in many cases more testing with bigger models.
Innovation:
- Encouraging Creativity: Depict how the lower cost and availability of SLMs support trial and error and innovativeness among designers.
- New Opportunities: Investigate the additional opportunities that SLMs open up, for example, novel applications and administrations that were already not doable.
Challenges with Large Language Models (LLMs)
Resource Intensity:
- Computational Demands: Stress the critical computational assets expected to prepare and run LLMs, which can be a hindrance for some associations.
- Energy Consumption: Examine the ecological effect of LLMs because of their high energy use, which raises manageability concerns.
Data and Bias:
- Data Quantity: Feature the gigantic measures of information expected to prepare LLMs, which can be challenging to source and make due.
- Inherent Biases: Make sense of how predispositions in preparing information can prompt one-sided yields, influencing the model’s decency and dependability.
Accessibility and Inclusivity:
- Cost Barriers: Discuss the significant expenses related with LLMs, which can keep more modest substances from getting to state of the art computer based intelligence innovation.
- Concentration of Power: Examine how the command over LLMs is in many cases gathered in the possession of a couple of enormous partnerships, possibly smothering development and variety in the field.
Ethical Considerations:
- Ethical Use: Address the moral problems presented by LLMs, for example, the potential for abuse in making misdirecting data or deepfakes.
- Regulatory Challenges: Notice the provokes in managing LLMs to guarantee moral and dependable use.
Technological Advancements in Small Language Models (SLMs)

Innovative Training Techniques:
- Transfer Learning: Examine how move gaining permits SLMs to use information from bigger models without the requirement for broad information.
- Sparse Data Techniques: Make sense of how SLMs can be prepared really even with restricted information, utilizing progressed calculations that attention on information effectiveness.
Model Optimization:
- Pruning and Quantization: Feature strategies like pruning, which diminishes the model size without critical loss of execution, and quantization, which decreases the accuracy of the model’s boundaries to accelerate derivation.
- Architecture Innovations: Discuss the improvement of new brain network models that are explicitly intended for SLMs to enhance execution.
Open Source Contributions:
- Community-Driven Development: Stress the job of the open-source local area in driving the progressions of SLMs, with cooperative ventures and shared assets.
- Accessibility of Tools: Notice how open-source instruments and structures support the turn of events and sending of SLMs, making them more available to engineers around the world.
Real-World Applications:
- Versatility in Deployment: Show the different utilizations of SLMs in certifiable situations, from chatbots to language interpretation administrations.
- Edge Computing: Talk about the appropriateness of SLMs for edge registering, where artificial intelligence handling is done locally on gadgets, decreasing inactivity and data transmission use.
Future Prospects:
- Potential for Growth: Hypothesize on the future headways in SLM innovation and the potential for considerably more modest and more productive models.
- Challenges Ahead: Momentarily address the difficulties that lie ahead in further streamlining SLMs for different applications.
Impact on Open Source and AI Community
Introduction:
- Start with an outline of the open-source development and its importance in the artificial intelligence local area.
- Lead into the conversation on how Little Language Models (SLMs) are impacting this space.
Open Source Philosophy:
- Collaboration and Sharing: Accentuate the basic beliefs of open source — coordinated effort, straightforwardness, and free circulation of programming.
- Community Empowerment: Examine how SLMs typify these standards by being more open to a more extensive scope of designers.
SLMs and Open Source Growth:
- Lowering Barriers: Feature how the lower asset necessities of SLMs empower more people and associations to add to artificial intelligence headways.
- Diverse Contributions: Discuss the variety of commitments that SLMs empower, prompting more inventive and fluctuated arrangements.
Democratization of AI:
- Widening Access: Make sense of how SLMs add to the democratization of computer based intelligence, making cutting edge innovations accessible to non-corporate substances.
- Educational Opportunities: Notice the job of SLMs in schooling, permitting understudies and analysts to explore different avenues regarding artificial intelligence without restrictive expenses.
Community-Driven Innovation:
- Rapid Prototyping: Portray how SLMs work with quick prototyping and trial and error, speeding up the speed of development.
- Open Source Projects: Give instances of open-source projects that have profited from the reception of SLMs.
Challenges and Opportunities:
- Sustainability: Address the maintainability of open-source projects fueled by SLMs, taking into account the harmony among development and asset the executives.
- Ethical Considerations: Examine the moral ramifications of boundless simulated intelligence access and the obligation of the open-source local area to maintain moral guidelines.
Conclusion
In conclusion, Little Language Models (SLMs) address a critical change in the artificial intelligence scene, offering a reasonable, productive, and open option in contrast to Enormous Language Models (LLMs). With their lower computational and energy prerequisites, SLMs are democratizing simulated intelligence, empowering a more extensive scope of designers to enhance and add to the field. The headways in SLM innovation are pushing the open-source local area forward as well as preparing for moral and dependable simulated intelligence improvement. As we keep on investigating the capability of SLMs, they stand to assume a significant part in store for simulated intelligence, encouraging a comprehensive climate where open-source progressions flourish and advantage society all in all.